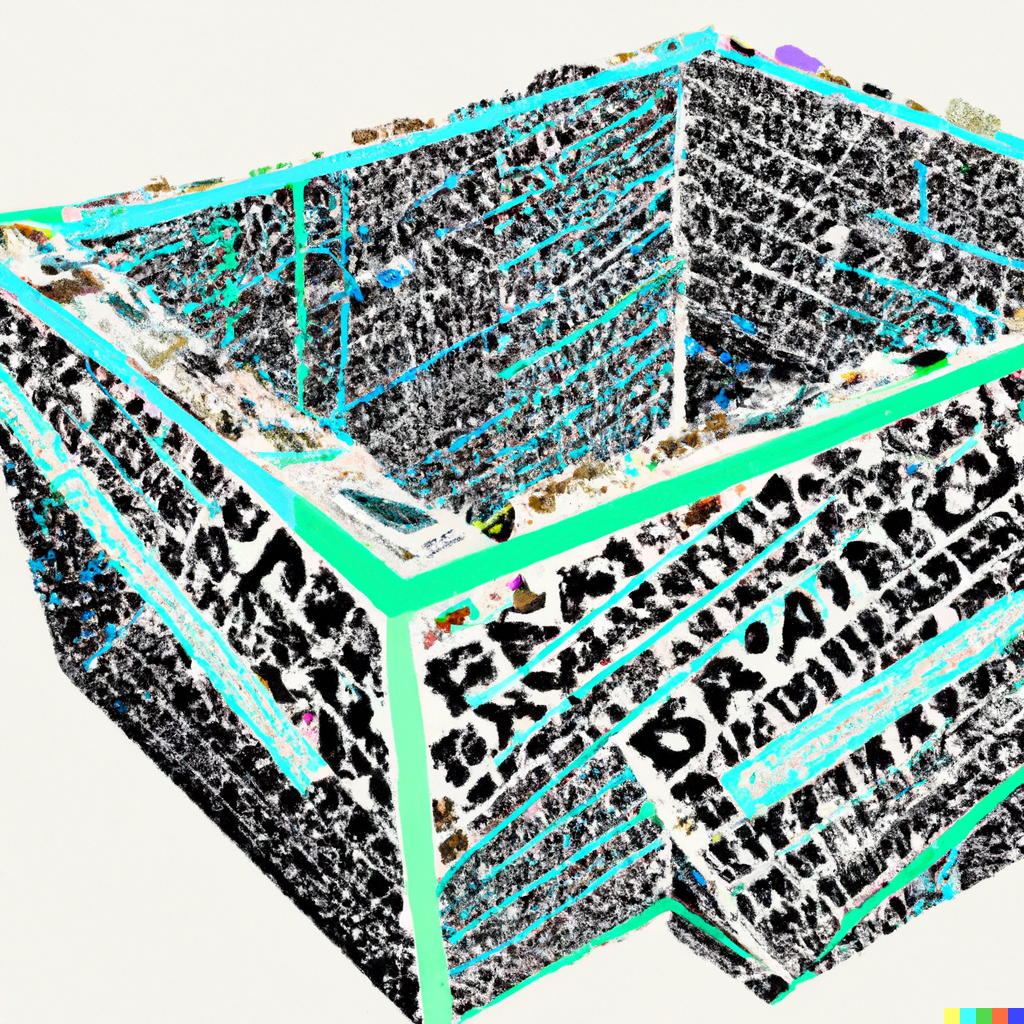
The Challenges of Banking Data Management: Unraveling the Data Pretzel
This report examines the challenges of banking data management – the complex intricacies, often called the ‘banking data pretzel.’ The inherent intricacy of this landscape emerges from the confluence of voluminous transaction data, disparate systems, varied data structures, and stringent regulatory requirements. This complexity, in turn, presents numerous challenges in data management, hindering the extraction of actionable insights, risk management, and regulatory compliance.
Let’s delve into cutting-edge technology and robust governance frameworks’ role in unraveling this banking data pretzel. Through an extensive analysis of existing technology solutions such as data warehousing, Big Data analytics, AI, machine learning, and cloud computing, we elucidate how they help simplify banking data. Moreover, we spotlight the pivotal role of robust governance frameworks in ensuring data integrity, quality, and regulatory compliance.
While technology solutions provide the tools to handle the data volume and complexity, a robust governance framework forms the bedrock for managing this technology. The right combination of both can significantly alleviate the challenges posed by the banking data pretzel.
We recommend that banks take a two-pronged approach to banking data management. Firstly, they should invest in state-of-the-art technology infrastructure that aligns with their unique requirements and capabilities. Secondly, they should concurrently establish and enforce a robust governance framework to ensure the effective use of this technology, maintain data integrity, and meet regulatory compliance.
Lastly, a proactive approach towards emerging trends in data management, like quantum computing and automated data governance, will keep banks at the forefront of this challenge. This strategy will empower them to untangle the existing data pretzel and remain resilient in future data complexities.
Resolving Banking Data Management Pretzel
Banks, as custodians of financial transactions and consumer financial data, have always been at the intersection of vast volumes of data. With the advent of digital transactions, online banking, and fintech innovations, the amount of data large banks manage has grown exponentially. This data deluge is quantitative and qualitative, ranging from customer demographic information to transactional data, risk data, market data, and more. Managing and deriving meaningful insights from this vast and diverse dataset has become a significant challenge for the banking industry, leading to what is often called the ‘banking data pretzel.’
The ‘banking data pretzel’ is a metaphor that accurately captures the complexities and intricacies of banking data management. It symbolizes the tangled mass of data that banks often grapple with due to disparate systems, diverse data structures, and many data sources. Add to this mix the stringent regulatory requirements that demand high levels of data quality, integrity, and transparency, and the result is a convoluted, hard-to-unravel data pretzel.
Legacy systems further exacerbate the problem many banks still operate on, leading to data silos and integration issues. The interoperability between various systems becomes challenging, and the resultant inconsistencies often lead to a lack of a unified view of the data. Furthermore, as banks engage with new technology platforms and digital interfaces, they continually add new layers to this complicated data pretzel.
However, understanding and managing this banking data pretzel is of paramount importance. Sound data management is the foundation of critical banking functions like risk management, customer relationship management, regulatory compliance, and strategic decision-making. Banks that effectively untangle their data can gain a competitive edge by delivering superior customer experiences, accurately assessing risks, making informed decisions, and ensuring regulatory compliance.
Technology and robust governance frameworks are essential in unraveling the banking data pretzel. Technology has shown immense promise in simplifying data management with solutions like data warehousing, Big Data analytics, AI, machine learning, and cloud computing. These technologies offer the ability to handle vast amounts of data, derive meaningful insights, and enhance operational efficiency.
Yet, technology alone is not the panacea. A robust governance framework is equally important to ensure data quality, accuracy, and integrity. Governance frameworks can help define clear data ownership, establish standard definitions, and implement effective data quality management procedures. They are crucial to regulatory compliance and can enhance trust in the data-driven decision-making process.
By examining technology solutions and governance frameworks, one can better understand how banks can effectively manage their data. It is essential for banking leaders, IT professionals, and policymakers in data management and governance.
The banking data pretzel represents one of the significant challenges facing the banking industry today. Untangling this pretzel requires a robust combination of advanced technology and stringent governance frameworks. We offer insights into this critical area. We intend to provide a pathway for banks to transform their data management practices, enhance operational efficiency, and gain a competitive edge in the increasingly data-driven banking landscape.
Understanding the Banking Data Pretzel
The term ‘banking data pretzel’ represents the intricate web of banking data that emanates from various sources, is stored in different formats, processed by diverse systems, and is governed by numerous regulations. This data sprawl introduces innumerable complexities and challenges that banks must grapple with daily.
At its core, the banking data pretzel has four main complexities. The first is data volume. Banks generate and collect massive amounts of data daily, from customer transactions and demographic details to market trends and risk data. This sheer volume of data is challenging to manage and process using traditional data handling tools and techniques.
The second complexity is data diversity. Banking data comes in various forms – structured and unstructured. While structured data such as account details and transaction records are relatively easier to manage, unstructured data like customer emails, call logs, and social media interactions are harder to process and analyze. Data collected from different channels can also lead to inconsistencies and duplications, further complicating the data landscape.
The third complexity is data integrity and quality. Ensuring that data is accurate, complete, timely, and consistent is a considerable challenge for banks. Disparate data sources and systems, manual data entry, and data integration issues often lead to errors and discrepancies in data. These errors can significantly impact the bank’s decision-making, risk management, and regulatory compliance.
Lastly, regulatory compliance is complex. Banks operate in a heavily regulated environment, and various laws and regulations dictate how they manage and handle data. Ensuring their data management practices comply with these regulations is a significant challenge. Additionally, regulatory requirements often demand high data transparency, integrity, and security levels, further complicating data management.
To illustrate, consider a large multinational bank with operations in several countries. This bank would deal with data from various sources – different departments, numerous customers, multiple channels, and different jurisdictions. This data would be in different formats and languages and must comply with various regional and global regulations. Additionally, the bank would likely use multiple systems and technologies to handle this data, leading to potential integration issues and data silos. This situation is a perfect example of a banking data pretzel – a tangled, complex web of data that is hard to manage and decipher.
The implications of the banking data pretzel are far-reaching. Ineffective data management can lead to poor decision-making, regulatory non-compliance, increased risk, and decreased customer satisfaction. For instance, inaccurate data can lead to incorrect risk assessments and potential financial losses. Similarly, failure to comply with data protection regulations can result in heavy penalties and damage the bank’s reputation.
Moreover, the inability to derive actionable insights from data can put banks at a competitive disadvantage. In an increasingly digital world, data-driven insights are crucial for understanding customer behavior, predicting market trends, and making strategic decisions. Banks that fail to untangle their data pretzel risk missing out on these insights, hindering their ability to innovate and compete effectively.
Understanding and managing the banking data pretzel is a critical challenge for the banking industry. The complex web of banking data, characterized by high volumes, diverse formats, integrity issues, and regulatory complexities, requires a robust combination of technology and governance. Banks that successfully untangle this data pretzel can transform it from a challenge into an opportunity, leveraging their data to gain a competitive edge, enhance operational efficiency, and ensure regulatory compliance.
The Role of Technology in Simplifying Banking Data Management
Technology has become essential to the banking industry, providing advanced tools and capabilities to handle the data deluge. As banks grapple with the banking data pretzel, technology offers a promising solution to simplify data, derive actionable insights, and enhance operational efficiency.
At the heart of these technology solutions is the ability to manage large volumes of data, process diverse data types, ensure data quality, and comply with regulatory requirements. Additionally, these technologies can automate manual tasks, reduce errors, and deliver real-time insights, transforming how banks manage their data.
One of the foundational technology solutions in this area is data warehousing. A data warehouse is a centralized repository where data from various sources is collected, integrated, and stored. This technology allows banks to break down data silos, ensure data consistency, and provide a unified view of their data. Banks can store vast amounts of historical data with a data warehouse, enabling them to perform complex queries and analyses. It forms the backbone of a bank’s data infrastructure, paving the way for more advanced analytics and data processing.
Big Data analytics is another critical technology in simplifying banking data. Banks can process large volumes of structured and unstructured data, extract meaningful patterns, and deliver actionable insights. Big Data analytics can help banks understand customer behavior, identify market trends, detect fraud, and make informed decisions. Hadoop and Spark are widely used for processing big data, handling petabytes, and delivering real-time insights.
Artificial Intelligence (AI) and Machine Learning (ML) have also emerged as powerful tools for managing banking data. These technologies can automate data processing tasks, detect anomalies, and predict trends. For instance, ML algorithms can analyze transaction data to detect fraudulent activities, significantly enhancing a bank’s risk management capabilities. AI can also be used in data cleansing and preprocessing, identifying and rectifying errors and inconsistencies in data, thereby improving data quality.
Moreover, AI and ML can generate deep insights into customer behavior, helping banks personalize their services and enhance customer experience. For example, ML algorithms can analyze customers’ transaction history, online behavior, and social media interactions to understand their preferences and needs. This information can then be used to offer personalized product recommendations, enhance customer engagement, and boost customer loyalty.
Cloud computing is another technology that is critical in simplifying banking data. Cloud platforms offer scalable, flexible, and cost-effective data storage and processing solutions. Banks can leverage the cloud to store large volumes of data, scale their data infrastructure per their needs, and access advanced data processing and analytics tools. Furthermore, cloud providers offer robust security measures and compliance tools, helping banks ensure data security and meet regulatory requirements.
Additionally, the cloud offers the advantage of accessibility. Banks can access their data from anywhere, anytime, enabling them to deliver real-time services and enhance operational efficiency. The cloud also facilitates collaboration, allowing different teams and departments to access and work on the same data, breaking down data silos and improving data consistency.
Technology offers a potent arsenal of tools to tackle the banking data pretzel. From data warehousing and Big Data analytics to AI, Machine Learning, and cloud computing, these technologies can help banks manage their data more effectively, extract meaningful insights, and enhance operational efficiency. However, technology is not a panacea. It must be complemented with a robust governance framework to ensure data quality, integrity, and regulatory compliance.
The Importance of Robust Governance Frameworks
While technology provides the tools to manage and simplify the banking data pretzel, a robust governance framework lays the foundation for effective data management. A robust governance framework defines the policies, procedures, and standards that govern how data is collected, stored, processed, and used within a banking institution.
A robust governance framework typically includes data ownership and stewardship, data quality management, privacy and security protocols, and regulatory compliance procedures. It provides a blueprint for managing data throughout its lifecycle, ensuring its quality, integrity, and compliance with regulations.
Data ownership and stewardship are crucial elements of a robust governance framework. Clear data ownership ensures that there is accountability for data quality and integrity. Data stewards are crucial in maintaining data, ensuring it adheres to established quality standards, and resolving data-related issues. They act as the data custodians, ensuring proper management and use.
Data quality management is another critical component of a robust governance framework. This involves establishing data accuracy, completeness, consistency, and timeliness standards. Data quality management tools can validate data, identify and rectify errors, and monitor data quality over time. Banks can make accurate decisions, mitigate risks, and ensure regulatory compliance by ensuring high data quality.
Data privacy and security protocols are also essential in a strong governance framework. These protocols define how data is protected from unauthorized access, breaches, and theft. They also dictate how customer data is handled, ensuring compliance with data protection laws and regulations.
Lastly, a robust governance framework incorporates regulatory compliance procedures. Given the heavily regulated nature of the banking industry, banks must ensure that their data management practices comply with various laws and regulations. A governance framework can help banks stay on top of these requirements, implement necessary controls, and maintain audit trails for regulatory reporting.
Banks that successfully implement robust governance frameworks offer tangible proof of their effectiveness. For instance, HSBC has implemented a global data governance framework that consistently manages data across its various businesses and regions. This framework has helped the bank improve its data quality, streamline regulatory reporting, and enhance its risk management capabilities.
Similarly, JPMorgan Chase has established a strong data governance framework for its broader data management strategy. The bank has a dedicated team of data stewards responsible for ensuring data quality and compliance with internal and external data standards. This approach has helped the bank improve its data quality, meet regulatory requirements, and deliver superior customer services.
A robust governance framework is as critical as technology in unraveling the banking data pretzel. It provides the structure, standards, and controls for managing data, ensuring its quality, integrity, and regulatory compliance. While technology provides the tools for managing and simplifying data, a robust governance framework ensures that this technology is used effectively and responsibly. Together, they form the foundation for effective data management in the banking industry.
Combining Technology and Governance Frameworks
The complexities of the banking data pretzel necessitate a combined approach that leverages both technology and governance frameworks. While technology provides the tools to handle vast amounts of diverse data, governance frameworks ensure that this data is dealt with effectively, responsibly, and in compliance with regulations.
Technology can greatly support robust governance frameworks. For instance, data quality management tools can automate validating, cleaning, and monitoring data, ensuring high quality. Similarly, data security technologies can help implement data privacy and security protocols, protecting data from unauthorized access and breaches.
Moreover, AI and machine learning can be leveraged to streamline regulatory compliance. These technologies can automate monitoring regulatory changes, implementing necessary controls, and maintaining audit trails. They can also automate the regulatory reporting process, ensuring accuracy and timeliness.
On the other hand, governance frameworks can guide the appropriate use of technology. They can set the standards for technology use, ensuring it is used responsibly and ethically. For instance, a governance framework can dictate how AI and machine learning are used, ensuring that they are used in a manner that respects privacy, ensures fairness, and avoids bias.
Additionally, governance frameworks can guide the selection and implementation of technology solutions. They can help determine which technologies suit a bank’s data management needs, ensuring that technology investments deliver the desired outcomes.
Several banks have demonstrated the success of this combined approach. For instance, Barclays has implemented a comprehensive data management strategy that combines advanced technology solutions with a robust governance framework. The bank uses big data analytics, AI, and machine learning to manage and analyze its data, supported by a powerful governance framework that ensures data quality, integrity, and regulatory compliance. This approach has enabled Barclays to improve its decision-making, enhance customer service, and ensure regulatory compliance.
Similarly, DBS Bank has successfully leveraged technology and governance to manage its data. The bank has a centralized data platform that uses advanced technologies like big data analytics and AI to collect and analyze its data. This technology infrastructure is supported by a strong governance framework that ensures data quality, security, and regulatory compliance. This combined approach has helped DBS Bank become a leading digital bank, delivering superior customer experiences and making data-driven decisions.
Combining technology and governance frameworks is crucial to unraveling the banking data pretzel. Technology provides the tools to manage and analyze data, while governance frameworks ensure that this data is collected effectively, responsibly, and in compliance with regulations. Banks combining these elements can transform their data management practices, gain a competitive edge, and deliver superior services in the increasingly data-driven banking landscape.
Future Outlook for Banking Data Management
As we look toward the future, several trends will likely shape how banks manage their data. These trends will influence technology solutions and governance strategies, reflecting the evolving demands of customers, regulators, and the broader market.
One trend to watch is the growing importance of real-time data. As customer expectations rise and competition intensifies, banks increasingly recognize the need for real-time data processing and analytics. This trend will likely spur technological advancements like in-memory computing and stream analytics, enabling banks to analyze and act on data in real-time.
Another trend is the increasing use of AI and machine learning in data management. While these technologies are already being used to some extent, their use will likely become more pervasive. AI and machine learning can automate data processing tasks, detect anomalies, and predict trends, helping banks improve their decision-making, risk management, and customer service.
In terms of governance, one future trend is the growing emphasis on data ethics. As banks leverage more advanced technologies like AI and machine learning, ensuring these technologies are used ethically and responsibly is becoming a priority. This trend is likely to lead to the development of more robust data ethics policies and frameworks.
We can also expect to see tighter integration between technology and governance. As the complexity of banking data continues to rise, the need for a cohesive approach that combines technology and governance is becoming increasingly apparent. This trend will likely lead to the developing integrated data management platforms that combine advanced technology capabilities with robust governance features.
Furthermore, regulatory technology, or RegTech, is anticipated to grow. It uses technology to enhance regulatory processes, and the rise in data volume and regulation complexity will likely drive the growth of RegTech solutions. This will help banks to meet their compliance requirements more efficiently and effectively.
These trends have several implications for banks and regulators. For banks, they underscore the need for ongoing investment in technology and governance. Banks must continually update their technology infrastructure and governance frameworks to meet evolving trends and demands. This may involve adopting new technologies, updating existing systems, and retraining staff to equip them with the necessary skills.
For regulators, these trends highlight the need for flexible and forward-looking regulation. As technology evolves, regulators must ensure that their regulations keep pace, protecting consumers and maintaining market stability without stifling innovation. This may require regulators to develop deeper technology expertise and adopt more flexible, risk-based regulatory approaches.
The future of banking data management will likely be characterized by rapid technological advancement, evolving governance requirements, and increasing regulatory scrutiny. Banks that stay ahead of these trends, investing in the right technologies and governance strategies, will be well-positioned to untangle their banking data pretzel and leverage it for competitive advantage.
Recommendations for Banking Data Management
In light of the challenges and trends discussed, several recommendations can be put forward to help banks unravel their data pretzels and harness the power of their data.
First, banks need to adopt a strategic approach to data management. Rather than treating data as an afterthought, banks should put data at the center of their strategic planning. This involves defining clear data management objectives, aligning these objectives with the bank’s overall business strategy, and ensuring that resources are allocated to meet these objectives.
Second, banks should invest in the right technology solutions. This involves identifying the technologies most suited to their data management needs, considering factors such as the volume and complexity of their data, data processing requirements, and budget constraints. Banks should prioritize flexibility, scalability, and integration capabilities when selecting technologies to ensure their technology infrastructure can evolve with their needs.
These technologies should be implemented in a phased manner to minimize disruption. Banks should start with pilot projects to test the effectiveness of the technology, learn from these pilots, and then scale up their implementation based on the lessons learned.
Third, banks should develop a robust governance framework. This framework should define the policies, procedures, and standards that govern how data is collected, stored, processed, and used. It should include data ownership and stewardship provisions, data quality management, data privacy and security, and regulatory compliance.
This governance framework should be communicated to all staff and enforced consistently. This may involve training staff on the framework, establishing a dedicated data governance team, and incorporating data governance responsibilities into staff job descriptions.
Fourth, banks should adopt a proactive approach to regulatory compliance. Rather than viewing compliance as a burden, banks should see it as an opportunity to enhance their data management practices. This involves staying abreast of regulatory changes, implementing necessary controls, and maintaining audit trails for regulatory reporting.
Finally, banks should cultivate a data-driven culture. This involves promoting data literacy among staff, encouraging data-driven decision-making, and rewarding data-driven innovation. By fostering a data-driven culture, banks can ensure that their data management efforts are not just a top-down initiative but a shared responsibility that permeates the entire organization.
Unraveling the banking data pretzel is a complex task that requires a strategic approach, the right technology, robust governance, proactive compliance, and a data-driven culture. Banks that adopt these recommendations will be well-positioned to manage their data effectively, derive actionable insights, and deliver superior services in the data-driven banking landscape.